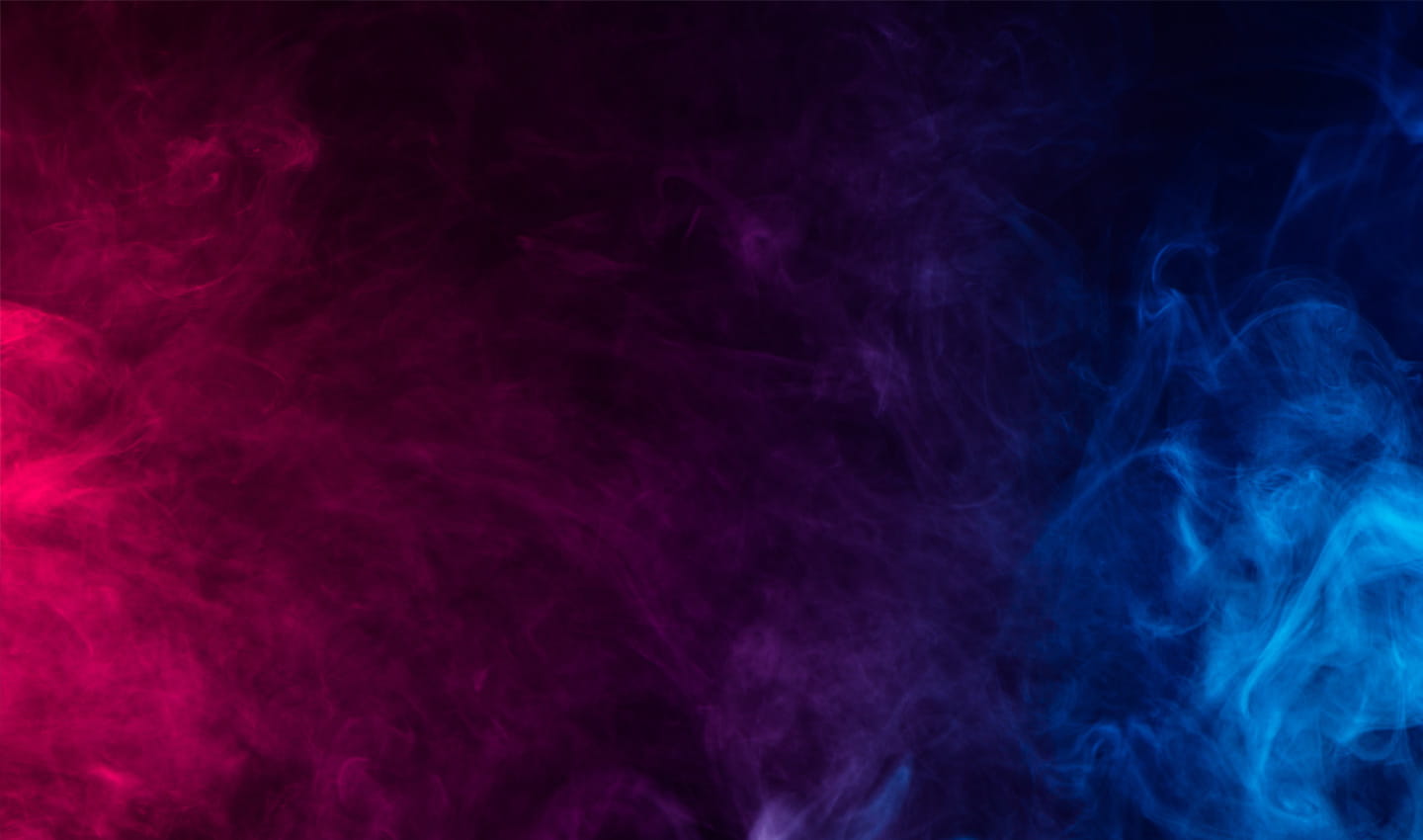
This domain name registration has expired.
If you are the domain owner, please contact support@epik.com to get the renewal taken care of.
The domain you are buying is delivered upon purchase.
Buy with confidence. Your purchase is secured by Epik.
Free WHOIS privacy, free forwarding, 24/7 Support are all standard.